Program Oncology
registration requested
Wednesday 26 June 2024
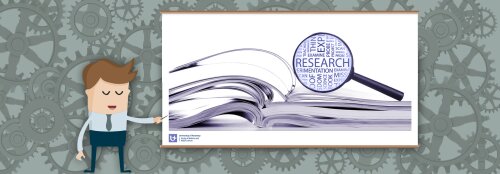
Location: room D.S.037
Campus Drie Eiken, Building S
Time:
13:00 - Daniel Flender
13:30 - Thomas De Kerf
+ network reception at 14:00
REGISTRATION
13:00 - Integrating Immunopeptidomics and RNA Sequencing for Neoantigen Discovery in NSCLC
by Daniel Flender
Non-small cell lung cancer (NSCLC) accounts for 80-85% of all lung cancer cases and remains a leading cause of cancer-related deaths worldwide. Despite significant advancements in immunotherapy, the current treatments still fall short largely due to high heterogeneity of tumors across patients. Therefore there is a pressing need for the discovery of new targets for novel immunotherapy approaches, for instance for the promising CAR T cell therapy. One such target is neoantigens—novel peptide sequences that arise from tumor-specific mutations and/or expression and that are presented by the major histocompatibility complex (MHC) on tumor cells. These antigens can than be used to develop new therapies such as T-cell therapy, therapeutic vaccines and others.
This study aims to identify highly specific neoantigens in NSCLC patients by combining patient-specific multiomic approaches, including immunopeptidomics and RNA sequencing. In immunopeptidomics, mass spectrometry is used to , identify immunopeptides presented by MHC on the cell surface, providing direct insight into the immunoepitope landscape.
In this study we used resection samples from 12 NSCLC patients, including both tumor and adjacent healthy tissue. RNA was extracted from these samples and sequenced to identify patient-specific mutations. We employed DeepVariant analysis to detect single nucleotide variants (SNVs) and insertions/deletions (INDELs), which were then used to create patient-specific FASTA databases. These databases were crucial for our subsequent immunopeptidomics analysis, as they enabled us to match the raw mass spectrometry data from each patient's tissue to their specific mutations using PEAKs and MSFragger software.
Our immunopeptidomics analysis extended to benign tissues from each patient, ensuring that the identified neoantigens were specific to the tumor and not present in healthy tissues. This step is vital to prevent potential autoimmunity in therapeutic applications. Additionally, RNA-Seq data allowed us to identify the HLA subtypes for each patient, which is helpful for further bioinformatic analysis and validation of the results. This analysis included predicting the binding affinity of the identified neoantigens to the patient's specific MHC subtypes, further validating their potential as true neoantigens.
Proteomic analysis was also integrated into our study. We included proteomics data from both tumor and benign tissues. A comparative analysis at the proteomic and immunopeptidomics levels provided deeper insights into the molecular pathways up or down regulated regulated in the tissuesand the tumor microenvironment.
Preliminary data from our study suggest that our immunopeptidomics approach is highly specific and capable of identifying tumor-specific and tumor-associated immunopeptides. The RNA sequencing data obtained so far have allowed us to generate patient-specific databases, setting the stage for identifying promising neoantigens.
In conclusion, we have established and applied an advanced immunopeptidomics platform to a cohort of NSCLC patients, analyzing both tumor and benign tissue samples. The creation of patient-specific databases enables us to pinpoint neoantigens that are unique to the tumor tissue, potentially paving the way for personalized immunotherapy treatments. The comparative analysis of proteomic and immunopeptidomics data between tumor and benign tissues enhances our understanding of the molecular dynamics within the tumor microenvironment. Our study underscores the potential of combining multiomic approaches to discover actionable neoantigens, which could significantly impact the development of targeted therapies for NSCLC.
13:30 - Artificial staining in pathology
by Thomas De Kerf
In clinical pathology and biomedical research, traditional histological staining, essential for tissue and cellular visualization, is resource-intensive and inaccessible in low-resource settings. Leveraging deep learning, we introduce a transformative approach: virtual staining. This technique enables the digital conversion of a single stained image into multiple stain types, significantly simplifying the staining process. This presentation will highlight the current research into virtual pathology and the potential of this technique.